Byung-Doh Oh (오병도)
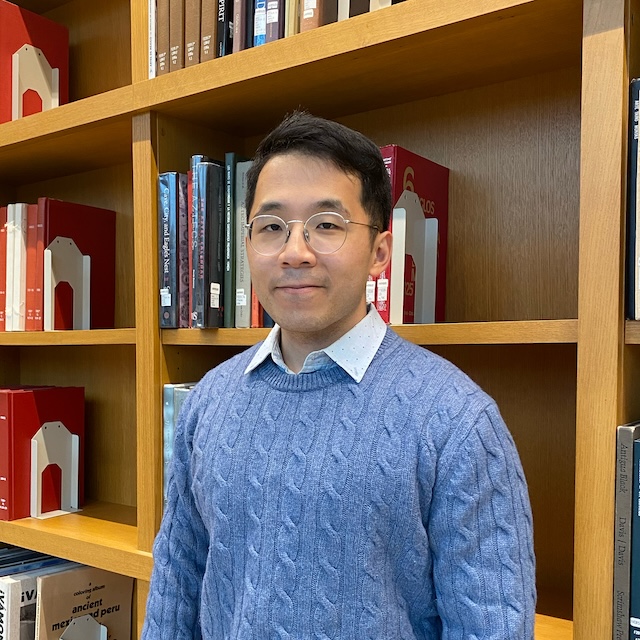
I am a Faculty Fellow at the Center for Data Science at New York University, where I collaborate with Tal Linzen. I received my PhD in computational linguistics from The Ohio State University, where I worked with William Schuler.
My work aims to advance our understanding of language processing in humans and machines by drawing on techniques from psycholinguistics and machine learning. I am particularly interested in developing computational models that capture the real-time processing behavior of human language users, and interpretability techniques for studying the predictions and representations of neural networks.